Research professors from Case Western Reserve University have unveiled a new quality-control tool for digital tissue imaging that flags low-quality images using a series of measurements and classifiers
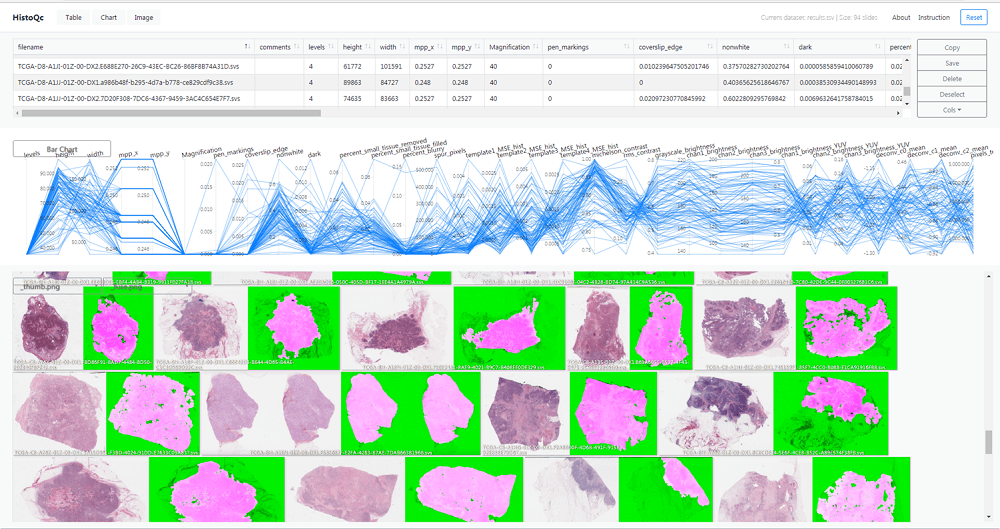
HistoQC programme (Credit: Andrew Janowczyk)
Using human tissue to detect cancer can cause problems for doctors when slides get marked or distorted – but new imaging software is able to separate faulty and clear samples for the first time.
Research professors from Case Western Reserve University, in Cleveland, Ohio, have created the HistoQC programme, which they say can digitally resample vast amounts of tissue images in order to sort faulty images from the high-quality sets for better diagnoses.
The team claims it is the first open-source quality-control review tool for digitised tissue imaging slides.
The technology, unveiled in the most recent edition of the Journal of Clinical Oncology Clinical Informatics, will be further developed with support from a three-year grant worth $1.2m (£930,000) from the National Cancer Institute.
Anant Madabhushi, biomedical engineering professor at the Case School of Engineering, believes it marks a significant move towards “a true democratisation of imaging technology.”
He said: “The idea is simple – assess digital images and determine which slides are worthy for analysis by a computer and which are not.
“This is important right now as digital pathology is taking off worldwide and laying the groundwork for more use of AI for interrogating tissue images.”
How can the quality of digital tissue imaging be distorted?
Current imaging technology is able to identify thousands of images per second.
However, it analyses digital images based on tissue slides that pathologists have been using for years as there are no go-to standards for preparing and digitising pathology slides, resulting in low-quality images mixing in with higher-quality slides.
Those slides consist of distortions that result in failure to identify tissues when viewed through a microscope, reducing the performance of sophistocated machine learning classifiers, such as disease diagnosis, and prediction of prognosis and therapy response.
The faults in quality of tissue imaging slides can be formed during its preparation and include issues such as air bubbles, smears, tissue folding and ragged cuts – known as “artefacts”.
This occurs in the tissue or even during the digitisation process, which may also cause blurriness and brightness problems.
Dr Janowczyk explained: “A microscope can’t focus on areas that have distorted quality, and it took me days to go through all those slides to manually identify and remove the bad ones.
“It was then that I realised we needed a faster, automated way to make sure we had only the good tissue slide images.”
How HistoQC helps pathologists identify low quality tissue images?
The HistoQC name derives from “histology” – the study of the microscopic structure of tissues – and “quality control.”
It can help clinicians make accurate diagnoses as the automated and quantifiable tool is incorporated with a series of measurements and classifiers, which can flag low-quality images that are difficult for doctors to read.
The system uses numerous image metrics and enhancements such as colour histograms, brightness and contrast.
It also features software for edge detectors, as well as “supervised classifiers to identify artefact-free regions on digitised slides”.
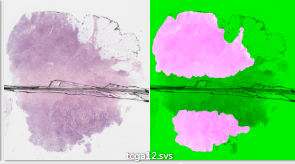
These regions and metrics are delivered by an interactive graphical user interface to the user through real-time visualisation and filtering.
The HistoQC software was found to be suitable for computational analysis more than 95% of the time.
As the application is “open source”, it is available for other companies or people to use, modify and extend. It can be accessed through an online repository.
To develop the software, Andrew Janowczyk, creator of HistoQC and assistant research professor in Center for Computational Imaging and Personal Diagnostics at Case Western Reserve University, analysed slides from the Cancer Genome Atlas.
He told Compelo: “Pathology slides have typically been reviewed by highly-educated humans who have been trained to be insensitive to the presence of staining variations and artefacts.
“As pathology departments are transitioning from glass slides to digital slides for interpretation, an opportunity for computer algorithms to aid in the analysis of these digital slides becomes a possibility.
“Unfortunately, computer algorithms are not naturally robust to the presentation of artefacts such as dust, cracks, smudges, pen-markings and over or under-staining of samples.
The Cancer Genome Atlas is a landmark cancer genomics programme housing more than 30,000 cancer tissue samples, from which Dr Janowczyk identified nearly 800 slides and found 10% had problems.
He added: “Our HistoQC tool was thus designed to help set standards and identify slides which may require reprocessing for accurate interpretation by algorithms.
“Taken as a pre-processing step for other algorithms, we anticipate specifying acceptable slide quality parameters ahead of time will improve downstream algorithmic performance.”