Radiologists can improve their methods to diagnose brain aneurysm using a new AI tool developed by medical experts and scientists at Stanford University
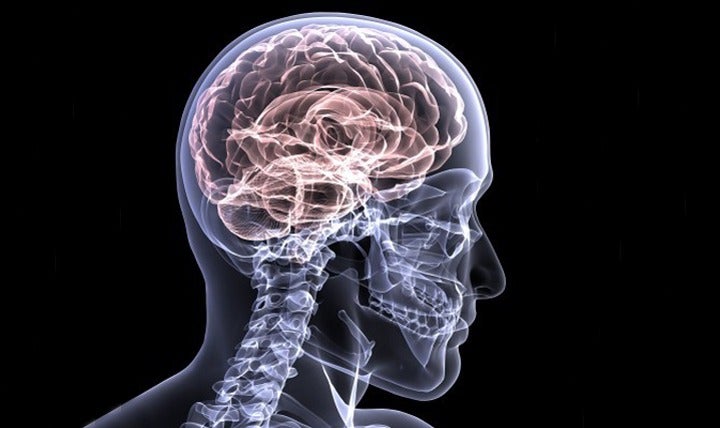
HeadXNet improved clinicians’ ability to correctly identify aneurysms (Credit: Healthmil)
An AI-powered tool that can assist radiologists diagnose brain aneurysms by highlighting the area affected has been developed by researchers at Stanford University.
Based on an algorithm called HeadXNet, the new AI tool enabled clinicians to identify six more aneurysms across 100 scans of the condition.
Detecting brain aneurysms – large bulges formed in blood vessels that can cause life-threatening health issues such as strokes and death – requires combing brain scans for signs, which means scrolling through hundreds of images.
Dr Kristen Yeom, an associate professor of radiology at Stanford University, said: “Search for an aneurysm is one of the most labour-intensive and critical tasks radiologists undertake.
“Given inherent challenges of complex neurovascular anatomy and potential fatal outcome of a missed aneurysm, it prompted me to apply advances in computer science and vision to neuro-imaging.”
How the AI algorithm to diagnose brain aneurysms was trained
Aneurysms come in many shapes and sizes and balloon out at tricky angles – some register as no more than a blip within the high definition succession of images.
The Stanford team used a training set of 611 computerised tomography angiogram head scans to outline clinically-significant aneurysms, and eight clinicians tested HeadXNet by interpreting 115 separate scans both with the algorithm and without it.
The automated detection tool led to improvements in sensitivity, mean accuracy and mean inter-rater agreement, although no significant changes were observed in mean specificity or time to diagnosis.
Christopher Chute, a Stanford graduate student in computer science, explained: “We labelled, by hand, every voxel – the 3D equivalent to a pixel – with whether or not it was part of an aneurysm.
“Building the training data was a pretty gruelling task and there was a lot of data.”
Following the training, the algorithm decides for each voxel of a scan whether there is an aneurysm present.
The result of the HeadXNet tool is the algorithm’s conclusions overlaid on the scan as a semi-transparent highlight, which allows radiologists to still examine the underlying image.
The algorithm doesn’t limit the scan to just identifying the presence of the condition, but also highlights the exact locations of the aneurysms to the clinicians.
In a study titled Deep Learning-Assisted Diagnosis of Cerebral Aneurysms Using the HeadXNet Model, details of the research were published in a medical journal JAMA Network Open, investigating the AI tool’s efficiency in helping clinicians interpret and identify aneurysms.
“There’s been a lot of concern about how machine learning will actually work within the medical field,” said Stanford graduate student Allison Park.
“This research is an example of how humans stay involved in the diagnostic process, aided by an AI tool.”