CINA-iPE is the first tool in the CINA Incidental suite of medical imaging solutions from the France-based Avicenna.AI
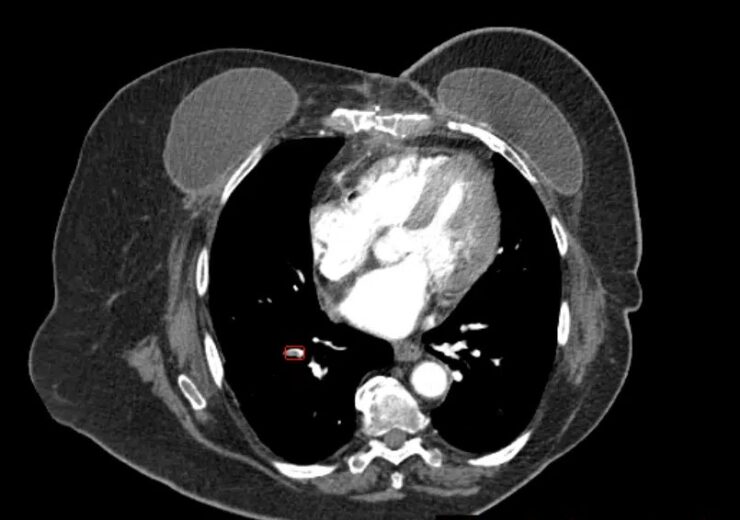
CINA-iPE is the first tool of Avicenna.AI in its new AI suite for incidental findings. (Credit: Avicenna.AI)
Avicenna.AI has launched CINA-iPE, a CE-marked artificial intelligence (AI) tool to analyse images from chest computerised tomography (CT) scans to detect the presence of incidental pulmonary embolism.
CINA-iPE is the first tool in the CINA Incidental suite of medical imaging solutions from the France-based Avicenna.AI. The new suite from the medical imaging company is intended for the detection of unsuspected pathologies on CT scans.
The CINA Incidental suite will be joined by the company’s existing suite CINA ER, which includes various tools for neurovascular and thoraco-abdominal emergencies.
The imaging firm said that these tools are US Food and Drug Administration (FDA)-cleared and CE-marked.
All the AI tools are said to be integrated seamlessly within clinical workflow and algorithm findings are automatically triggered and reported through the systems already in use by radiologists.
Avicenna.AI co-founder and CEO Cyril Di Grandi said: “If pathologies are visible on a CT scan, the technology now exists to detect them – helping clinicians reduce time-to-treatment and save lives.
“Our CINA Incidental suite helps healthcare professionals detect incidental findings in patients receiving imaging for entirely different health conditions, improving patient care and outcomes.
“The launch of CINA-iPE is the first step in a new direction for Avicenna.AI. Pulmonary embolism is a dangerous, life-threatening condition, and with CINA-iPE we hope to increase the number of patients identified with incidental PE and help improve their outcomes.”
The company offers healthcare AI solutions that leverage deep learning to spot, identify, and quantify life-threatening pathologies from CT medical images.
Avicenna.AI’s products are claimed to automatically identify and prioritise emergency situations within seconds, evaluate their severity, and inform radiologists without delay using machine learning technology.