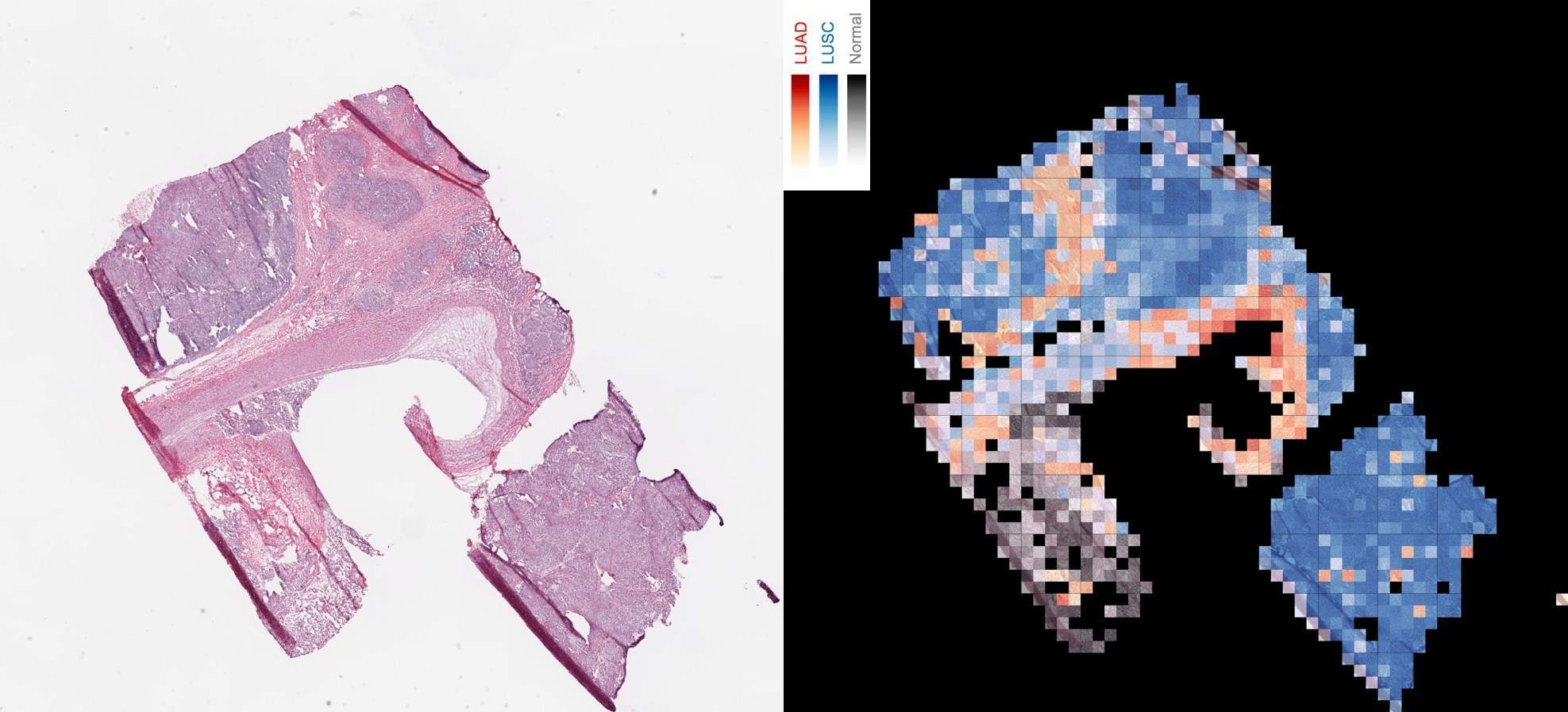
The study found that a type of artificial intelligence (AI), or machine learning program, could distinguish with 97% accuracy between adenocarcinoma and squamous cell carcinoma.
In the study, it was also found that the AI program was able to analyze from images and determine whether abnormal versions of six genes linked to lung cancer including EGFR, KRAS and TP53 were present in cells, with an accuracy that ranged from 73 to 86% depending on the gene.
These genetic changes or mutations can often cause abnormal growth associated with cancer, but can also change a cell’s shape and interactions with its surroundings, providing visual clues for automated analysis.
The researchers stated that about 20% of patients with adenocarcinoma are known to have mutations in gene epidermal growth factor receptor or EGFR, which could be treated with approved drugs. But, one of the major issues in determining the specific genetic mutations is that it can take several weeks for the test results to come in.
NYU School of Medicine Department of Pathology associate professor and senior study author Aristotelis Tsirigos said: “Delaying the start of cancer treatment is never good. Our study provides strong evidence that an AI approach will be able to instantly determine cancer subtype and mutational profile to get patients started on targeted therapies sooner.”
In the study, the NYU team developed statistical techniques that gave the program the ability to ‘learn’ how to get better at a task but without being told exactly how. Such programs use rules and mathematical models that enable decision-making based on data examples given to them, with the program getting “smarter” as the amount of training data grows.
New AI approaches, inspired by nerve cell networks in the brain use complex circuits to process information in layers, where each step feeds information to the next and assigns more or less importance to each piece of information.
The team trained Google’s Inception v3, a deep convolutional neural network, to analyze slide images from The Cancer Genome Atlas, a database of images where cancer diagnosis had been completed.