A new research has found that artificial intelligence (AI) can dramatically cut time needed to process abnormal chest x-rays.
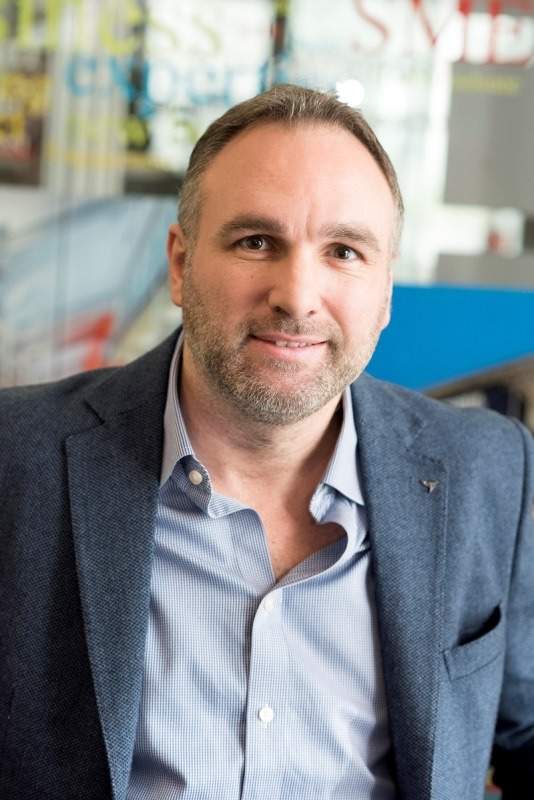
Image: University of Warwick, WMG Professor Giovanni Montana. Photo: Courtesy of University of Warwick.
Chest X-rays are routinely performed to diagnose and monitor a wide range of conditions affecting the lungs, heart, bones, and soft tissues.
With the new AI technology, the abnormal chest X-rays with critical findings are expected to receive an expert radiologist opinion within less time.
Researchers from WMG at the University of Warwick, working with Guy’s and St Thomas’ NHS Hospitals, have carried out researches to develop the AI system. As part of the research, they have extracted half a million anonymised adult chest radiographs (X-rays).
The researchers have developed an AI system for computer vision which is capable of recognizing radiological abnormalities in the X-rays in real-time and suggest how quickly these exams should be reported by a radiologist.
The research team has developed a Natural Language Processing (NLP) algorithm while building the AI system, to read a radiological report, understand the findings mentioned by the reporting radiologist, and automatically infer the priority level of the exam.
The team produced a large volume of training exams for the AI system that allowed it to understand visual patterns in X-rays which were predictive of their urgency level.
The research team was led by Professor Giovanni Montana, Chair in Data Science in WMG at the University of Warwick, has found that normal chest radiographs were detected with a positive predicted value of 73% and a negative predicted value of 99%.
At a speed that is understood as abnormal radiographs with critical findings are to be prioritized for receiving an expert radiologist opinion much sooner than the usual practice.
University of Warwick, WMG Professor Giovanni Montana said: “Artificial intelligence led reporting of imaging could be a valuable tool to improve department workflow and workforce efficiency. The increasing clinical demand on radiology departments worldwide has challenged current service delivery models, particularly in publicly-funded healthcare systems.
“It is no longer feasible for many Radiology departments with their current staffing level to report all acquired plain radiographs in a timely manner, leading to large backlogs of unreported studies.”
The research results are published in Radiology journal in a paper entitled “Automated triaging and prioritization of adult chest radiographs using deep artificial neural networks.” on 22 January 2019.