Study published in Breast Cancer Research shows how Deep-Learning of over 650 breast cancer digital pathology images and Omics data can be used together to unlock precise mechanisms of therapy resistance
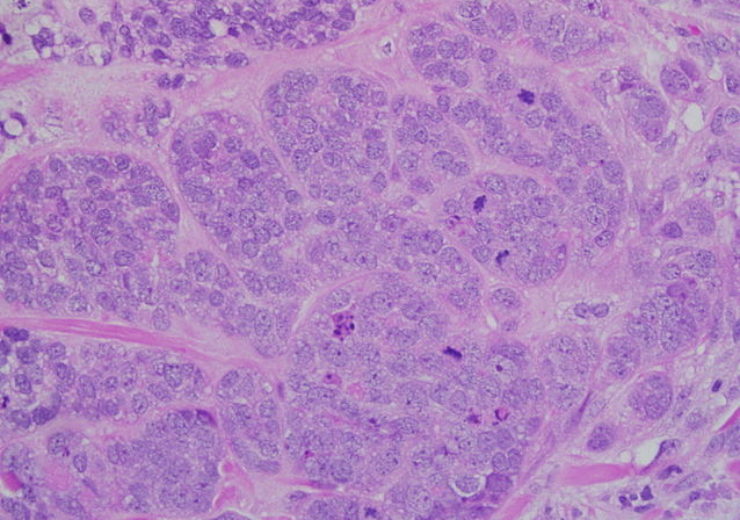
https://commons.wikimedia.org/wiki/File:Invasive_Ductal_Carcinoma_40x.jpg
NantOmics, the leader in molecular analysis, and NantHealth, a next-generation, evidence-based, personalized healthcare company, announced today the publication of a peer-reviewed study in Breast Cancer Research, a Springer Nature journal, on a novel AI technique in breast cancer. The study reports on a novel deep-learning system of digital pathology images and omics data used together to more precisely identify mechanisms of therapy resistance.
“Our analysis builds on our breadth of advanced machine learning technologies to better support providers in therapeutic decision-making and to improve the capabilities of the underlying molecular analysis technology platforms that we use at NantHealth and NantOmics”
Tweet this
NantOmics scientists trained a deep-neural network on diagnostic slide images from 443 breast tumors that had previously undergone PAM50 subtyping to classify patches of the tumor images into four major molecular subtypes of breast cancer (basal-like, HER2-enriched, luminal A, and luminal B). The algorithm was then validated and demonstrated the capability to establish accurate breast cancer sub-typing in 222 samples from a retained set of tumors. By focusing the neural-network’s attention on cancer-rich regions in the diagnostic images, this deep-learning algorithm identifies patient biopsies that are a mixture of different molecular subtypes, a classification that is less definable from molecular pathology techniques. Patients with heterogeneous biopsies such as mixtures of basal-like and luminal disease have a different survival profile than patients with homogeneous disease, and may potentially benefit from a more tailored therapy regimen.
“Breast cancer can be subtyped into at least five distinct disease-types with very different prognoses and responses to therapy. These subtypes are characterized as clinically important, yet are typically only achievable by RNA expression profiling,” Dr. Patrick Soon-Shiong, MD, Chairman and CEO of NantHealth explained. “With this AI technique we achieved 87 percent accuracy rate in detecting which patients had basal-like breast cancer (i.e., triple negative breast cancer), one of the most aggressive subtypes,” he said.
“Our analysis builds on our breadth of advanced machine learning technologies to better support providers in therapeutic decision-making and to improve the capabilities of the underlying molecular analysis technology platforms that we use at NantHealth and NantOmics,” said Soon-Shiong. “Through the advances of machine vision and augmented intelligence, we have developed a rapid and cost-effective deep-learning technique to unlock the rich information in readily-available diagnostic slide images to define subtypes concordant with their underlying molecular designations.”
Breast Cancer Research, a Springer Nature journal, is an international, peer-reviewed online journal publishing original research, reviews, editorials and reports in breast cancer. Breast Cancer Research is published by BMC, an open access publisher that produces over 250 scientific journals and according to its website, is “the highest ranked breast cancer focused title in the field.”
Breast cancer with minimal tubule formation, marked pleomorphism, and prominent mitoses. (Credit: Wikipedia.org/Difu Wu.)
Source: Company Press Release