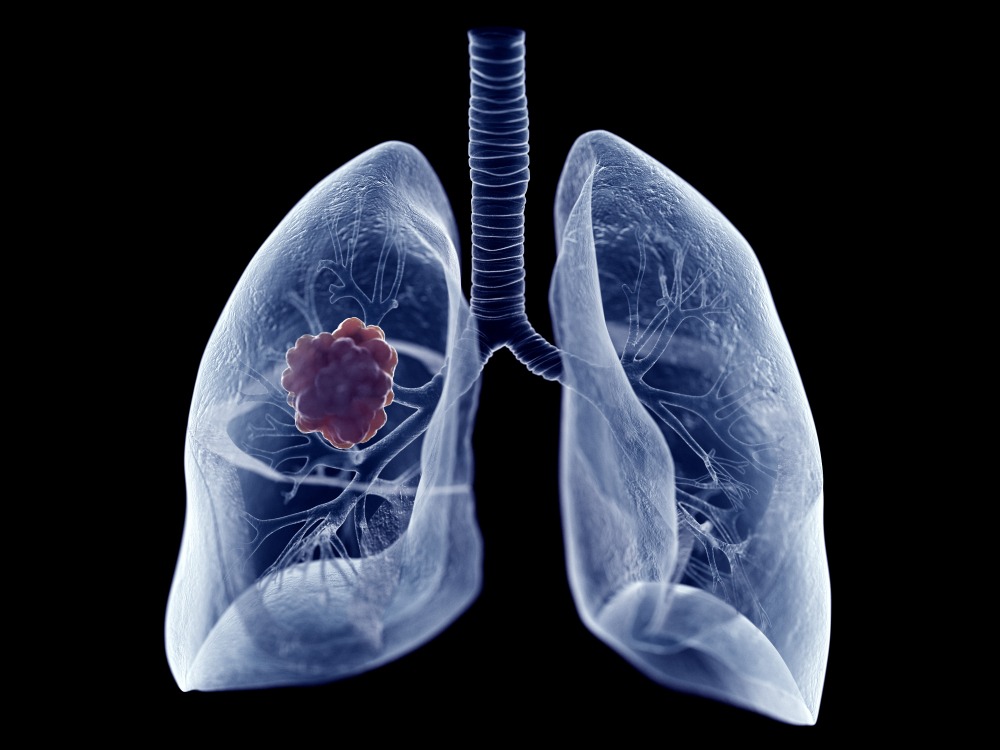
Lung cancer is the leading cause of cancer death worldwide, and one barrier to early detection is the challenge of determining which pulmonary nodules will remain benign – but an AI prediction tool could help doctors to foresee malignancy.
Researchers from the Diagnostic Image Analysis Group at Radboud (DIAG) University Medical Center (RUMC) in Nijmegen, the Netherlands, developed a deep learning algorithm for malignancy risk estimation of pulmonary nodules detected at low-dose screening CT scan.
When they evaluated it by pitting its predictive capabilities against experts in the field of radiology and pulmonology, they found it performed with equal accuracy, meaning it could be a valuable way to triage patients by risk.
“The algorithm may aid radiologists in accurately estimating the malignancy risk of pulmonary nodules,” said the study’s first author, Kiran Vaidhya Venkadesh, a PhD candidate with the DIAG at RUMC.
“This may help in optimising follow-up recommendations for lung cancer screening participants.”
The study was published in the journal Radiology
Lung cancer risk and chest CT
Low-dose chest CT is used to screen people at a high risk of lung cancer, such as longtime smokers.
It has been shown to significantly reduce lung cancer mortality, primarily by helping to detect cancers at an early stage when they are easier to treat successfully.
While lung cancer typically shows up as pulmonary nodules on CT images, most nodules are benign and do not require further clinical workup.
Accurately distinguishing between benign and malignant nodules is therefore crucial to catch cancers early, which is why there’s a research imperative to create AI-based tools that can assist physicians.
Study methodology and findings
For the new study, researchers developed an algorithm for lung nodule assessment using deep learning, an AI application capable of finding certain patterns in imaging data.
The researchers trained the algorithm on CT images of more than 16,000 nodules, including 1,249 malignancies, from the National Lung Screening Trial.
They validated the algorithm on three large sets of imaging data of nodules from the Danish Lung Cancer Screening Trial.
The deep learning (DL) algorithm delivered excellent results, outperforming the established Pan-Canadian Early Detection of Lung Cancer model for lung nodule malignancy risk estimation.
In an editorial accompanying the study, Martin C Tammemägi, PhD of Brock University who developed the Pan-Can model, wrote: “In the Danish validation cohort, the PanCan model discriminated very well, with an AUC of 0.90. However, the DL algorithm performed significantly better (AUC, 0.93).
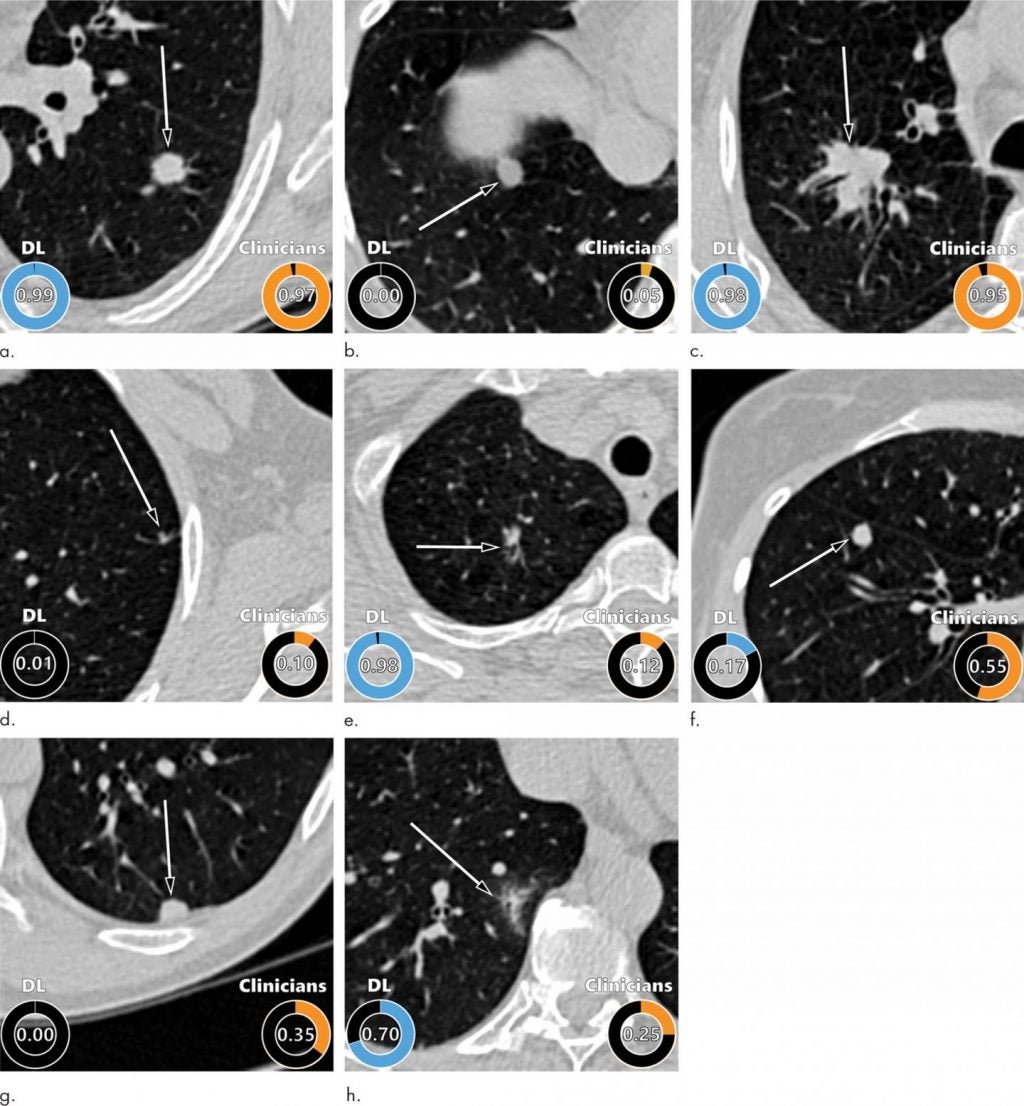
“It should be appreciated that it is difficult to move AUCs incrementally upward at these stratospheric levels.”
The DL algorithm also performed comparably to 11 clinicians, including four thoracic radiologists, five radiology residents and two pulmonologists.
Senior author Colin Jacobs, PhD, assistant professor in the Department of Medical Imaging at Radboud University Medical Center in Nijmegen said: “As it does not require manual interpretation of nodule imaging characteristics, the proposed algorithm may reduce the substantial interobserver variability in CT interpretation.
“This may lead to fewer unnecessary diagnostic interventions, lower radiologists’ workload and reduce costs of lung cancer screening.”
Next steps for AI lung cancer prediction
The researchers plan to continue improving the algorithm by incorporating clinical parameters like age, sex and smoking history.
They are also working on a deep learning algorithm that takes multiple CT examinations as input.
The current algorithm is highly suitable for analysing nodules at the initial, or baseline, screening, but for nodules detected at subsequent screenings, growth and appearance in comparison to the previous CT are important.
Dr. Jacobs and colleagues have developed other algorithms to reliably extract imaging features from the chest CT related to chronic obstructive pulmonary diseases and cardiovascular diseases.
They will be investigating how to effectively integrate these imaging features into the current algorithm.
In terms of how soon the DL algorithm could be used in clinical practice, Tammemagi’s editorial suggested it could be a while.
“Although this study shows promising results, it provides only the first steps in a long but necessary process,” he wrote.
“Many additional important steps must be completed before the DL algorithm can be widely accepted into screening practice.
“Although the DL algorithm appears to demonstrate potential use for lung cancer screening in radiologic practice, as with all models (including the PanCan model), it must undergo multiple iterations of external validation regardless of whether it is on the basis of regression or machine learning.”