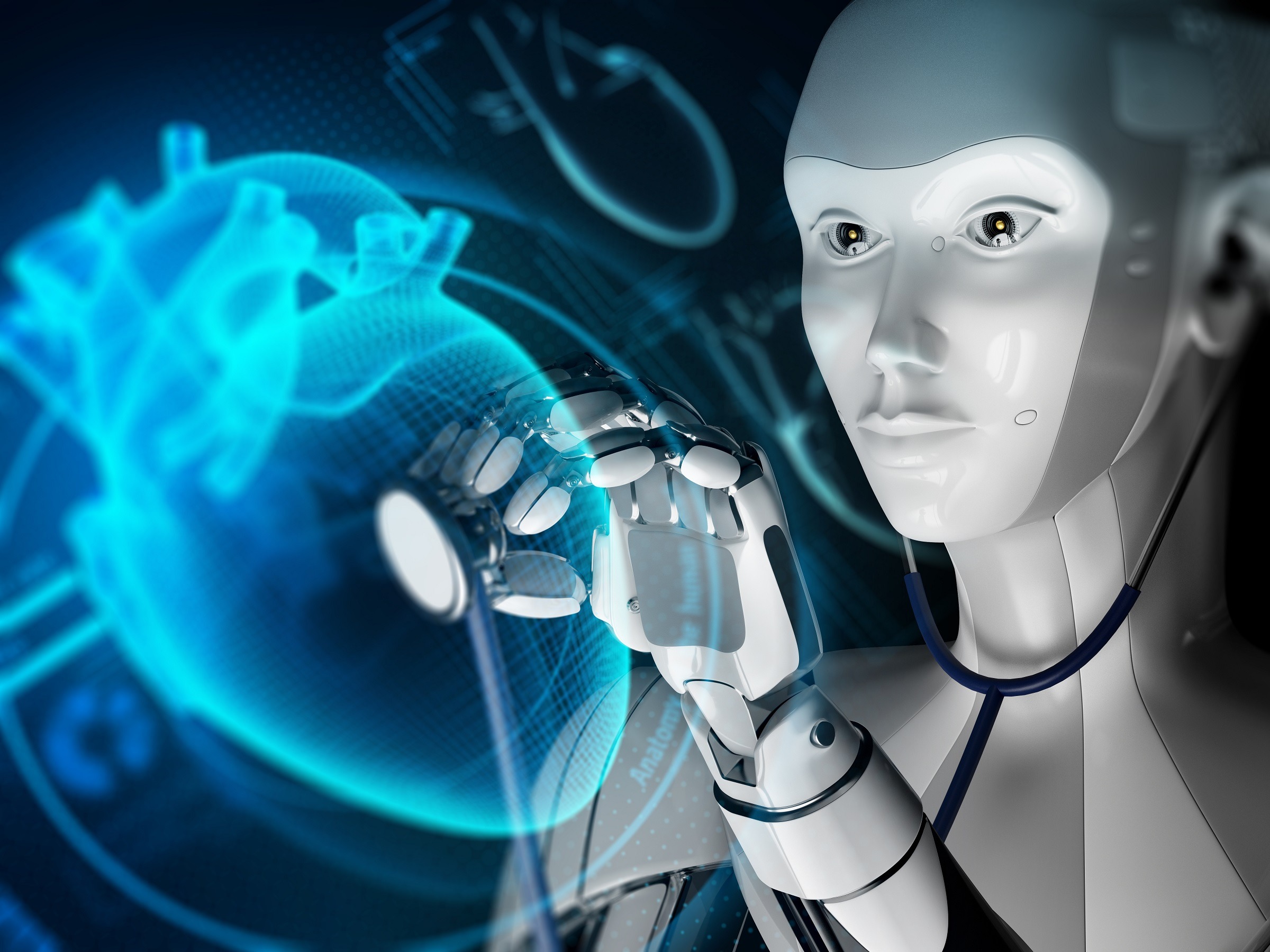
The rapid adoption of AI in healthcare to tackle Covid-19 may result in underlying data biases, and issues stemming from a lack of regulation, according to an analyst.
Artificial intelligence (AI) and machine learning (ML) have been adopted and integrated into the healthcare industry more quickly than ever before over the course of 2020 – largely due to the potential these technologies have in early SARS-CoV-2 detection, contact tracing, and even vaccine development.
Analytics firm GlobalData is anticipating this will lead the market for AI and ML to almost double in value – from $29bn last year to $52bn in 2024.
However, GlobalData medical device analyst Kamilla Kan also believes that, while this could be beneficial for the healthcare sector, it will be difficult for the industry to avoid the negative impacts resulting from underlying biases in data.
“Without strong policies and procedures to prevent bias in ML algorithms, there is a possibility that underlying biases in training data, and existing human biases, can be embedded into these algorithms,” she added.
“In the healthcare industry, when a patient’s life is on the line, biased ML algorithms could result in potentially serious consequences.
“For instance, some algorithm designs could ignore how numerous factors, such as sex, gender, age or the presence of other preliminary diseases, impact the current state of health.
“Understandably, many healthcare specialists are concerned that AI- or ML-powered algorithms could negatively influence current patient care.
“Currently, the FDA regulatory framework is not designed to handle adaptive algorithms and, without proper regulation, AI- or ML-powered algorithms could be trained on one demographic and used on a different one – which will produce biased and improper results.”
Underlying biases that may affect AI adoption in healthcare
According to GlobalData’s Global Emerging Technology Trends Survey 2020, more than 75% of companies believe AI has played a role in helping them survive the Covid-19 pandemic.
Despite these real-world benefits, the training data used to teach AI systems can include biased human decisions, or reflect historical or social inequalities – even when variables like gender, race and sexual orientation have been removed.
These biases can then inadvertently become ‘baked in’ to the algorithms used by AI or ML systems.
Biases can also be created by flawed data sampling, in which certain groups are incorrectly represented in the training data.
An online article published last year in the Harvard Business Review suggested that, based on academic research, two actions need to be taken to minimise AI bias moving forward – accelerating existing processes for addressing these biases, and taking advantage of the ways AI can be used to improve on traditional human decision-making.
But, with AI and ML now being adopted rapidly out of necessity during the coronavirus pandemic, it remains to be seen if healthcare systems and medical device companies will be able to prioritise these safeguards.