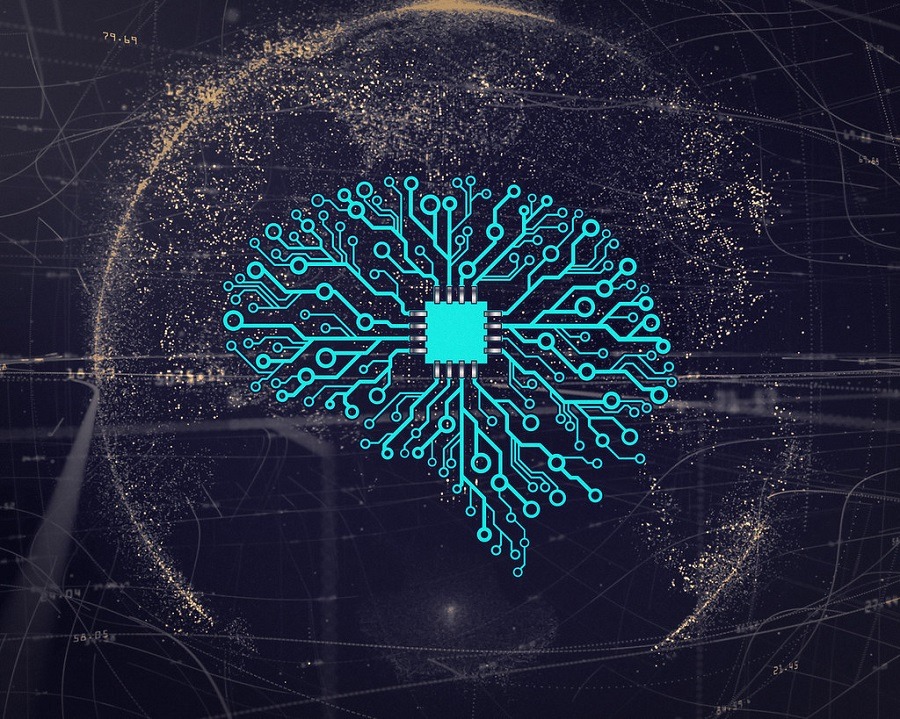
Machine learning decision models capable of predicting which patients diagnosed with depression might need further treatment have been developed by teams at healthcare research organisation Regenstrief Institute and Indiana University.
The algorithms were based on a wide range of patient-level diagnostic, behavioural and demographic data, including clinic visit history retrieved from the Indiana Network for Patient Care, a statewide health information exchange.
Designed to integrate into existing clinical workflows, research scientist Dr Suranga Kasthurirathne developed algorithms for the entire patient population at Eskenazi Health located in Indianapolis, Indiana, as well as several different high-risk groups.
He said: “By creating models for different patient populations, we offer health system leaders the option of selecting the best screening approach for their needs.
“Perhaps they don’t have the computational or human resources to run models on every single patient. This gives them the option to screen select high-risk patients.”
How machine learning can help patients with severe depression
Globally, the World Health Organisation estimates that depression affects about 350 million people and is the leading cause of disability currently costing America $210bn per year.
A study published in a journal of Social Psychiatry and Psychiatric Epidemiology revealed the US loses almost $5,524 per working person each year due to workers who come to work when they are depressed but are unable to be as productive.
An additional $390 per working person each year is lost because of absences related to the condition.
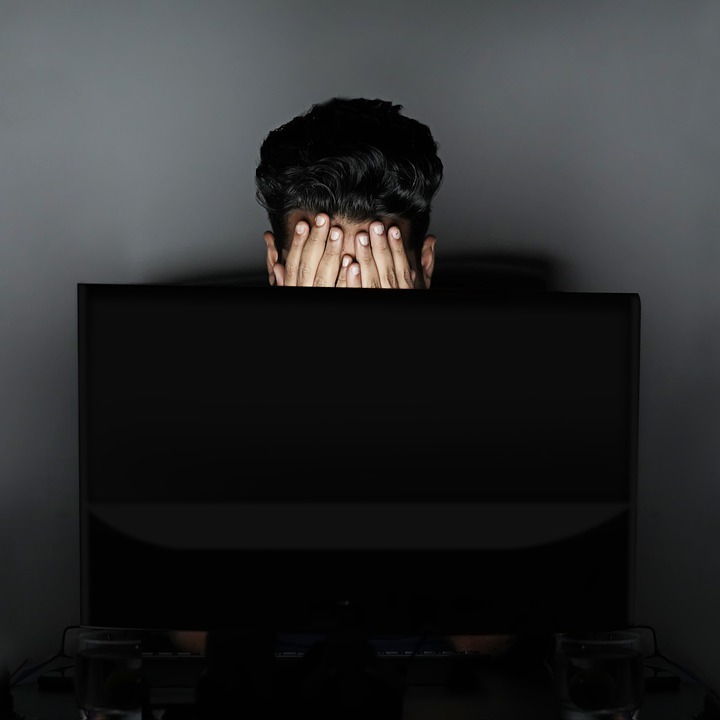
From genetic predisposition and biological factors to hormone imbalances, depression may emerge in a patient for a number of reasons.
There are also a number of risk factors that can trigger depressive episodes, such as anxiety and other mental health conditions, trauma, and some prescription medications.
With so many variables at play, there is no single treatment for the condition.
Dr Kasthurirathne said: “Our goal was to build reproducible models that fit into clinical workflows.
“This algorithm is unique because it provides actionable information to clinicians, helping them to identify which patients may be more at risk for adverse events from depression.”
Collecting biometric data could enable deep learning algorithms to find trends in behavioural and emotional patterns, helping doctors identify patients who have a high suicide risk.
Dr Kasthurirathne said: “Primary care doctors often have limited time, and identifying patients with more severe forms of depression can be challenging and time consuming.
“Our model helps them help their patients more efficiently and improve quality of care simultaneously.”
He explained that the algorithms are created to help primary care doctors identify patients with severe depression that requires advanced care from mental healthcare specialists.
Titled Identification of patients in need of advanced care for depression using data extracted from a statewide health information exchange: A machine learning approach, the study was published in the peer-reviewed Journal of Medical Internet Research.
The team of researchers are now working to incorporate social determinants of health — the economic and social conditions that influence individual and group differences in health status — into these models.