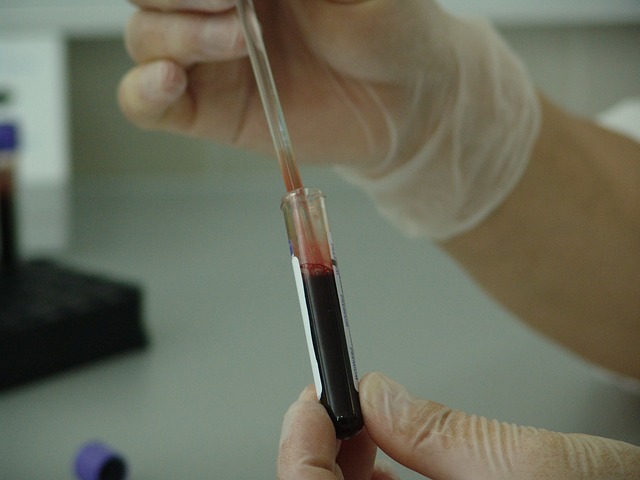
The VOCAL test is a liquid biopsy that combines mass spectrometry, artificial intelligence and machine learning to analyse tumour-associated changes in circulating glycoproteins.
The trial is a prospective, international, multi-centre, observational study that involves collection of de-identified samples and data from 1,200 women, in both the US and abroad.
The study is aimed at validating ovarian cancer-specific glycoproteomic signatures in the blood based on histologically confirmed malignancy status of the mass.
The primary endpoint of the study is to evaluate predictive values of the VOCAL test, and the secondary outcome is to analyse the relationship between glycoproteins and survival rates among women with ovarian cancer.
According to the American Cancer Society, ovarian cancer ranks fifth in cancer deaths among women, accounting for more deaths than any other cancer of the female reproductive system.
InterVenn BioSciences CEO Aldo Carrascoso said: “There is so little we understand about ovarian cancer that the primary course of treatment for women with a tumor in their ovaries is the partial or total removal of the organs and sometimes even the uterus.
“Through VOCAL, we hope to help physicians and patients better select a course of treatment most appropriate for their disease through an easy blood test that results in an improved quality of life.”
Glycoproteomics is an emerging field that integrates proteomics and glycomics, for better biomarker and target discovery, which also produces large amount of data that takes months to analyse.
InterVenn said that it would leverage mass spectrometry, hi-res characterization of post-translational modifications, and artificial intelligence to streamline and expedite the process.
In December 2018, InterVenn received an institutional investment of £7.5m from biotech investor Genoa Ventures, and was participated by True Ventures, Amplify Partners, Boost VC, and Prado SV.
The company’s approach combines the analysis of glycoproteomics using mass spectrometry with data science and artificial intelligence to quickly spot potentially actionable and clinically relevant information for conditions including cancers, autoimmunity, fibrosis, and neurodegeneration.