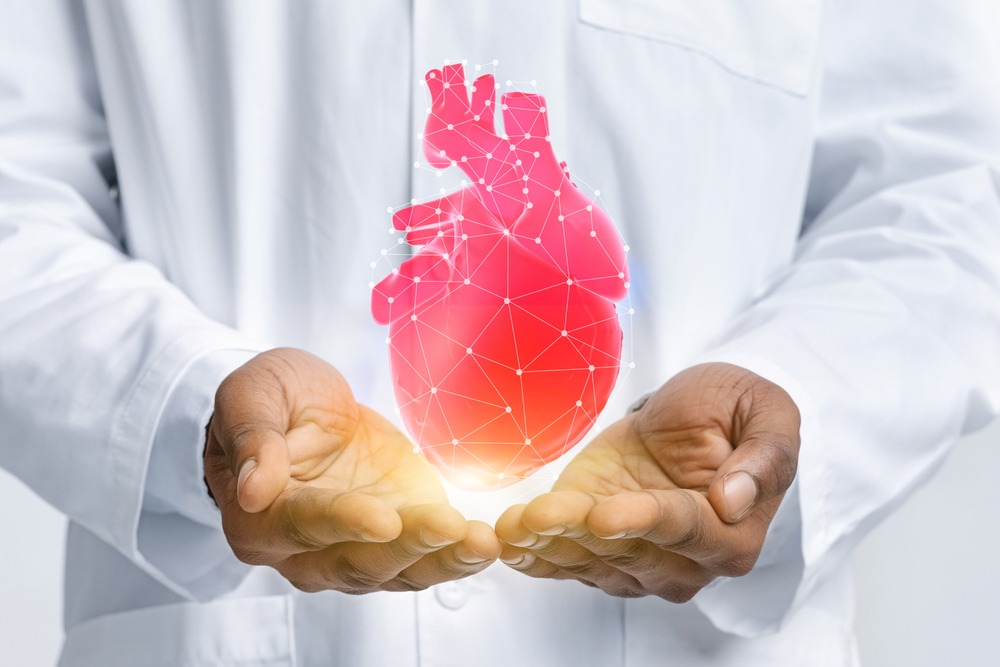
Coronary heart disease is the number one global killer, and one tell-tale sign of it developing is calcium deposits in the coronary arteries – but Harvard researchers may have found a way to use AI for more accurate cardiovascular risk prediction.
Typically, coronary artery calcification can be seen and measured with a heart-imaging test, like a CT scan.
But due to the lack of radiological expertise, time and specialised equipment available in hospitals, it’s not realistic for every patient to be given their coronary artery calcium score – a tool used to assess the risk of a heart attack or stroke in the next five to ten years.
That’s why researchers from Harvard Medical School affiliate Brigham and Women’s Hospital and the Massachusetts General Hospital’s (MGH) Cardiovascular Imaging Research Center (CIRC) teamed up to develop and evaluate a deep learning system that could lead to better detection of calcification, so that less patients fly under the radar.
“In theory, the deep learning system does a lot of what a human would do to quantify calcium,” said Roman Zeleznik, MSc, data scientist and lead author of the study conducted by the Artificial Intelligence in Medicine (AIM) Program at the Brigham and Harvard Medical School.
“Our paper shows that it may be possible to do this in an automated fashion.”
Study co-author Michael Lu, MD, MPH, director of artificial intelligence at MGH CIRC, emphasised the role AI could play not just in the prediction of risk, but also in personalising treatment of cardiovascular disorders.
“This is an opportunity for us to get additional value from these chest CTs using AI,” he said.
“The coronary artery calcium score can help patients and physicians make informed, personalised decisions about whether to take a statin.
“From a clinical perspective, our long-term goal is to implement this deep learning system in electronic health records, to automatically identify the patients at high risk.”
The researchers validated the AI system using data from more than 20,000 individuals, and called the results, which were published in the journal Nature Communications “promising”.
Study supporting AI for better cardiovascular risk prediction
The team began by training the deep learning system on data from the Framingham Heart Study (FHS), a long-term asymptomatic community cohort study.
Framingham participants received dedicated calcium scoring CT scans, which were manually scored by expert human readers and used to train the deep learning system.
The deep learning system was then applied to three additional study cohorts, which included heavy smokers having lung cancer screening CT (NLST: National Lung Screening Trial), patients with stable chest pain having cardiac CT (PROMISE: the Prospective Multicenter Imaging Study for Evaluation of Chest Pain), and patients with acute chest pain having cardiac CT (ROMICAT-II: the Rule Out Myocardial Infarction using Computer Assisted Tomography trial).
All told, the team validated the deep learning system in over 20,000 individuals.
According to the researchers, the automated calcium scores from the deep learning system “highly correlated” with the manual calcium scores from human experts.
The automated scores also independently predicted who would go on to have a major adverse cardiovascular event, like a heart attack.
Udo Hoffmann, MD, director of MGH CIRC, who was the principal investigator of CT imaging in the FHS, PROMISE and ROMICAT studies, emphasised the inclusion of three National Heart, Lung, and Blood Institute-funded high-quality image and outcome trials strengthens the generalisability of these results to clinical settings.
Could deep learning technology enter clinical settings?
Identifying people at risk of cardiovascular diseases (CVD) is a cornerstone of preventative cardiology – but according to one 2019 paper, predictive models are “typically based on a limited number of predictors with sub-optimal performance across all patient groups”.
The authors were looking beyond indicators of coronary heart disease, but even in this particular area there are biomarkers beyond calcification that can be put through a model to predict the risk of a cardiovascular event caused by this disease, like age, gender, smoking status and blood pressure.
As for the clinical relevance of the research from Zeleznik and colleagues, having a tool validated on a large study cohort would undoubtedly be a boon to hospitals, and if other risk factors like those identified above could be integrated into such an AI system, it may be possible to catch coronary heart disease, among other cardiovascular diseases, before they cause the death of a patient.
As the Harvard and MGH researchers have made the algorithm behind their deep learning system open source, it could be a good place to start in achieving this ambition.