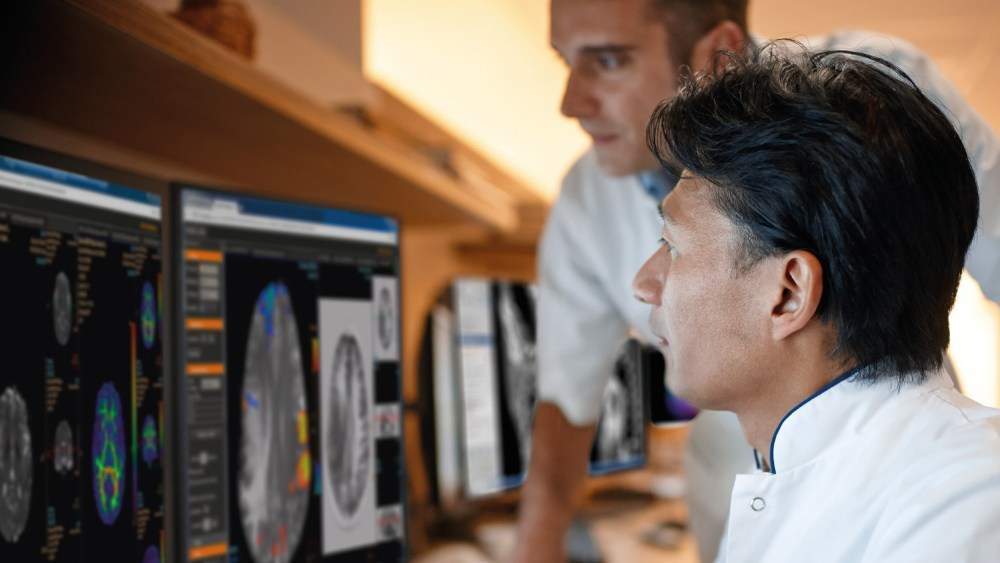
Philips’ IntelliSpace Discovery research platform is used by over 50 hospitals and academic institutions globally to develop radiology applications for rendering, segmentation, and quantification.
The platform is being utilized for research and clinical validation and subsequently the tools/applications can be deployed into the radiology workflow on the Philips IntelliSpace Portal.
The IntelliSpace Discovery 3.0 open platform intends to support radiologists in their clinical and translational research.
Powered by Philips HealthSuite, the platform offers radiologists comprehensive data analytics in medical imaging.
Philips said with the introduction of IntelliSpace Discovery 3.0, the platform now provides research applications and tools for radiologists to aggregate, normalize and anonymize data, which can be visualized and annotated to ‘train’ and validate deep learning algorithms.
The algorithms can then be easily deployed as plug-in apps into the research workflow to analyze new datasets and help facilitate clinical research in radiology, oncology, neurology and cardiology.
Philips chief innovation & strategy officer Jeroen Tas said: “Together with our customers we’re enabling research in adaptive intelligence with the goal to create solutions that augment healthcare professionals and improve patient care and efficiencies of care delivery, both inside and outside of the hospital.
“AI is the connective tissue to seamlessly integrate data and technology to enable precision diagnosis. At RSNA 2018 we’re showing how AI is laying the foundations for solutions of the future.”
The main components of IntelliSpace Discovery include front-end applicatons, study management, machine learning, clinical research, and research services.
Philips said integration with existing clinical infrastructure provides access to vendor agnostic and multi-modality data-sets, and research advanced visualization tools allow data to be prepared and processed for AI training with existing AI tools.
The fully scalable infrastructure contains a vendor neutral research archive for structured and unstructured data, which can be used to upload and process data.
The machine learning research data and deployment platform will receive batch processing in a scalable computing environment to support iterative development and validation.
The clinical research AI assets and capabilities will include multi-parametric enablement, segmentation and annotation, tumor quantification and stratification, deep learning networks and classification.